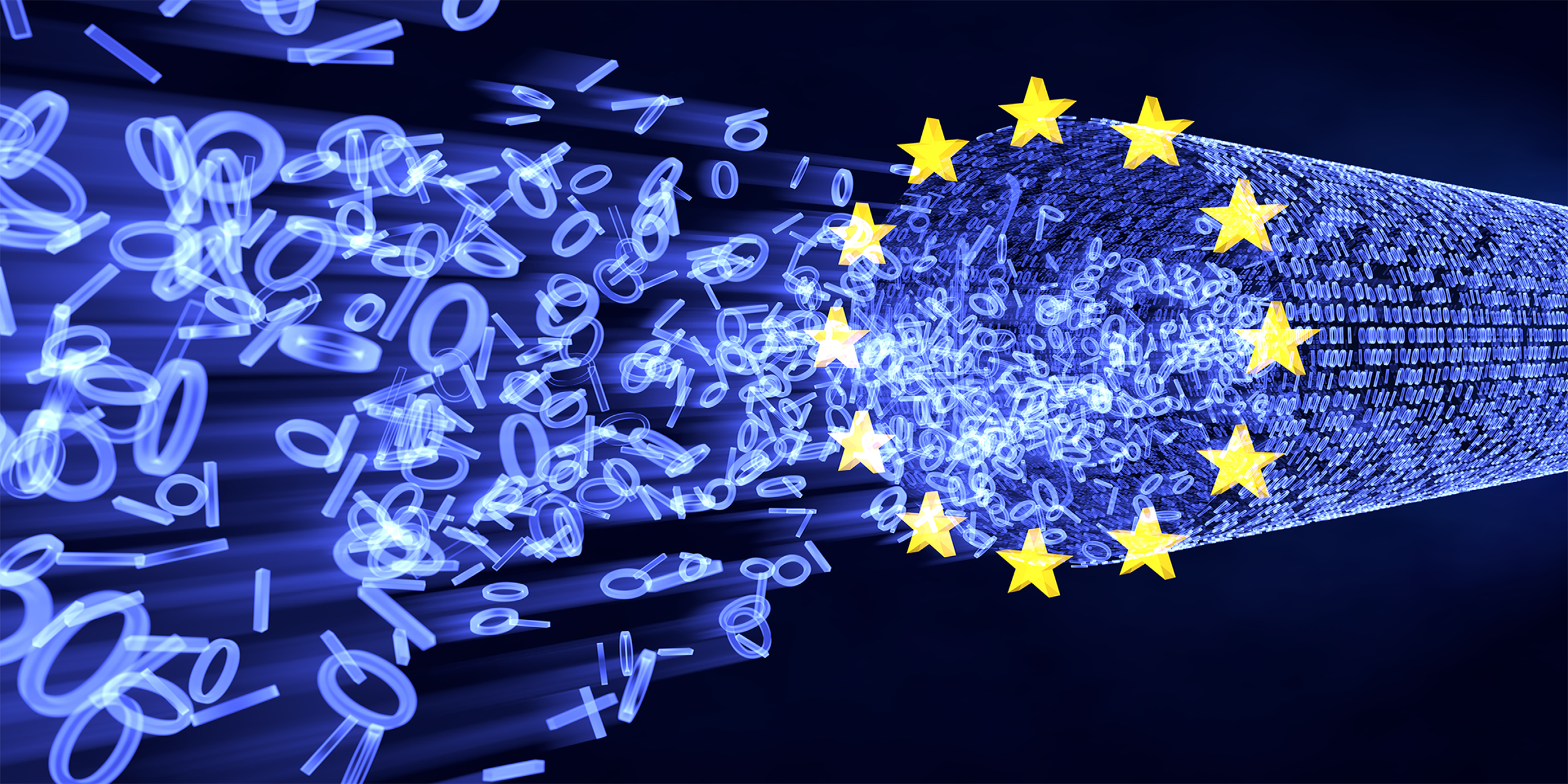
Data Classification Trends To Keep in Mind in 2018
This article was co-written by Kris Lahiri, Data Protection Officer and Dawid Balut, Egnyte Architect.
There are quite a few data challenges and trends we’ll see in 2018 and years to come. We’re producing and consuming more content than ever before. And we don’t see it slowing down anytime soon which is a good thing because this often means we’re innovating and producing a great deal of creative work.
- We must manage data volumes growing at a very fast pace. Data classification challenges at this scale are fairly new. If you’re a company that’s processing large datasets coming from hundreds to thousands of sources, you can’t reliably do it manually. Manually doesn’t work because it won’t scale, and in some cases doing it manually may be a privacy violation. When GDPR takes effect on May 25th, privacy violations will translate into much bigger consequences than the ones companies are used to. That’s the reason why we should work on building highly secure and well performing autonomic systems which can operate efficiently without human access to the data.
- Companies have a hard time identifying all the places where they process and store their data. This means they can’t reliably assess and classify it. Some companies don’t take into consideration legacy datasets which not only spoils the statistical analysis but also leaves unsecured data behind. Assets inventory is a never-ending problem of the security industry and data classification follows the patterns we’re used to seeing in other fields of information security for decades. As companies and their data volumes grow, we can see it becoming an increasingly severe problem, especially under the GDPR which in many cases doesn’t allow businesses to store data that is not being used for a specific purpose.
- We need sophisticated machine learning and big data analytics to come into play given the needs for data analysis and operating on anonymized data sets. Sadly, that’s easy to get wrong, and with data protection, it doesn’t matter how many files you got right because it takes one improperly secured file to compromise and fail the customer. That’s why it’s recommended for most companies to use a trusted vendor who can help get it right from the beginning. The future of data classification lies in the hands of companies that can competently put machine learning and artificial intelligence to use. Implementing practical solutions using machine learning on an enterprise level is complicated, and it requires a staff that is highly competent in developing data processing platforms.
- New regulations enforce data processing from companies have a higher level of security - which creates a demand for new types of auditing and monitoring tools as well as diligent security assessment to ensure there is no place where a user’s privacy is being violated. It also creates a need for modification of existing data classification mechanisms and algorithms that need to be now adjusted to comply with new data security standards as those imposed by GDPR. These systems must be able to encrypt, backup, and process the data with respect to the preservation of privacy while storing them in the appropriate data silos with safety controls.
We can definitely see more companies starting to realize the need to help corporations specialize in building sophisticated data classification solutions. Most companies should not offload unrealistic expectations to their IT teams and not expect them to develop improbable solutions. It's impractical to have demanding expectations for in-house that require immense manpower which big software companies possess and take years to develop and work out.