Enhancing Data Security with AI
Let’s jump in and learn:
Data security presents a host of challenges with increasingly sophisticated cyber attacks, including ransomware and phishing, putting sensitive data at constant risk. Internal threats, often stemming from unauthorized access or misuse of data by employees, also present notable risks. Additionally, compliance with evolving data protection regulations is both complex and time-consuming. As organizations undergo digital transformation and expand operations in the cloud and through the usage of Internet of Things (IoT) devices, securing data in those environments becomes even more challenging.
Artificial intelligence (AI) is quickly becoming a core component of data security. Using AI in data security, organizations are able to analyze data from disparate sources to detect patterns and usual activity that can indicate malicious activity. These systems also amplify threat detection capabilities with early warning notifications and automation.
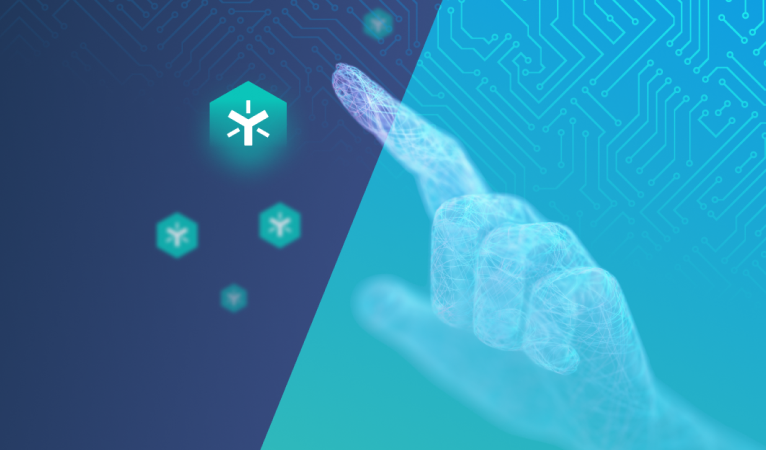
Understanding AI in Data Security
AI uses machines running algorithms and computational models to mimic human cognitive functions. AI in data security has become nearly mandatory. With AI in data security, systems can ingest and interpret large quantities of data at unparalleled speeds, resulting in early and accurate identification of security threats. The importance of AI in data security continues to grow as cyber threats become ubiquitous, persistent, and stealthy, and traditional security measures often fall short.
The addition of AI in data security fills gaps, providing broad coverage and more precise threat detection. AI in data security provides predictive analysis capabilities that allow organizations to anticipate future threats based on historical data and take targeted and proactive defensive measures. Additionally, AI in data security can automate responses to detected threats, helping to reduce response times and minimize potential damage.
Key Functions of AI in Data Security
Variants of AI perform a variety of functions, such as:
- Identifying patterns in vast data sets
- Interpreting natural (i.e., human) language
- Learning from experience
- Making predictions
- Perception
- Reasoning
- Solving problems
Underlying Technologies that Power AI in Data Security
Computer vision
Computer vision is used by AI in data security to provide advanced capabilities for interpreting and understanding visual information.
- Video surveillance for physical security - for analyzing video footage in real-time to detect and report suspicious activities.
- Automated event detection uses advanced algorithms to differentiate between routine activities and potential security threats in sensitive areas, such as data centers and corporate offices.
- Enhanced monitoring of physical spaces uses video that surveils the spaces to detect unauthorized access or suspicious activities (e.g., forced entry and loitering), such as unauthorized access to server rooms with systems that house sensitive data.
- Image analysis for identifying security breaches - for identifying indicators of potential security breaches found in visual information (e.g., sensitive video and documents).
- Breach documentation techniques analyze images to detect and document changes in the physical environment that might indicate a security breach, such as alterations made to digital images that may indicate an attempt to mask a security breach.
- Digital watermarking can be analyzed to detect illicit copying or alteration of digital documents and images through digital watermarking techniques and steganalysis, which detects hidden information within digital media.
- Facial recognition for access control - to ensure that only verified, authorized people can access physical and digital spaces where sensitive data is stored.
- Multi-factor authentication incorporates facial recognition as a verification factor to enhance security by adding a biometric layer that is difficult to forge or bypass.
- Secure access systems compare a live capture or digital image of a person’s face with the stored faceprint to verify their identity.
Machine learning algorithms
Various machine learning algorithms are used for AI in data security.
- Supervised learning algorithms - for classification and regression tasks.
- Fraud detection learns from historical fraud patterns and uses regression models to assess the risk level based on various indicators.
- Spam and phishing filters analyze email content, headers, and sender information to identify and filter out spam and phishing attempts based on characteristics identified in training datasets.
- Threat detection uses supervised learning algorithms that are trained on labeled datasets to classify activities as either normal or malicious.
- Unsupervised learning - for clustering and anomaly detection.
- Anomaly detection uses unsupervised learning algorithms that analyze data without prior labeling to detect activities that deviate from normal network behavior but do not match any known threat patterns (e.g., signs of zero-day attacks).
- Behavioral analysis tools cluster similar data points to identify unusual user behavior, even when the malicious activity does not match any known signature.
- Reinforcement learning - for adaptive security measures where the system learns to make decisions based on the reward or penalty it receives for its actions.
- Adaptive threat response can dynamically adjust security measures based on the outcome of previous actions.
- Network security policy optimization can optimize network security policies by continuously learning from network traffic patterns and threat incidents and automatically adjusting firewall rules, intrusion detection system settings, and other security policies.
- System hardening is used to test and improve system defenses by simulating attack scenarios continuously.
Natural language processing (NLP)
A subset of AI, security systems use NLP to interpret and analyze human language to detect and respond to security threats.
- Analysis of text-based threats, such as phishing messages
NLP techniques analyze the content of emails and identify phishing attempts based on the language, tone, context, and patterns of the messages. This includes detecting malicious intent, urgency cues, and spoofing tactics that are not always apparent through traditional spam filters. Advanced NLP models can understand the context and subtleties of language, making them effective in spotting sophisticated phishing schemes. - Language translation for global threat intelligence
NLP-powered language translation enables the automatic translation of threat intelligence, alerts, and security updates from various languages. This ensures that organizations worldwide can share and access vital security information rapidly, without language barriers. - Sentiment analysis for detecting insider threats
Sentiment analysis, a branch of NLP, can monitor and analyze communications within an organization to detect changes in sentiment or emotional tone that may indicate disgruntlement or malicious intent amongst employees.
How AI Enhances Data Security
AI brings data security to new heights, particularly with its capabilities in threat detection and prevention. Cybersecurity threats continuously evolve, advancing in sophistication and frequency, necessitating even more powerful solutions to combat them. AI in data security provides a number of capabilities that are required for effective threat detection and prevention, including the following.
Automated threat detection and response
AI excels in detecting anomalies and patterns indicative of potential threats. With AI in data security, vast amounts of information can be analyzed and filtered to efficiently detect threats that are embedded in the normal flow of data. Once a threat is detected, AI in data security systems can initiate automated responses to contain and mitigate such threats. This includes automatically isolating affected systems, blocking malicious IP addresses, and applying patches to vulnerabilities. The speed of AI-driven responses stops malicious activity quickly to minimize potential damage or data comprises.
Behavior analysis for anomaly detection
AI algorithms are adept at learning what normal behavior looks like for users and devices within an organization’s network, creating a behavioral baseline. Activities that deviate from this baseline, such as unusual login times or data access patterns, can be flagged for review to stop insider threats and external threat actors, including zero-day attacks and advanced persistent threats (APTs).
Continuous learning
Machine learning models in AI-powered data security systems continuously learn from the information they process, which allows them to adapt responses to emerging and evolving threats. These systems are also regularly updated with information from recent attacks to improve their threat detection capabilities over time.
Proactive threat detection
With machine learning and data analytics, AI in data security solutions can predict potential vulnerabilities and future attack vectors by analyzing trends and patterns revealed in historical data and real-time inputs.
Real-time monitoring and analysis
AI in data security tools can monitor network traffic and system activities in real time, analyzing data from various sources to detect suspicious activities. This can prompt automated responses to mitigate risks.
Scalability
For most organizations, the volume of data generated by users and machines continuously expands at an unrelenting pace, as does the complexity of networks. AI in data security solutions scales seamlessly to meet these increasing demands without compromising the speed or accuracy of threat detection and other security protections.
AI Applications in Data Security
The following are several widely used applications of AI in data security:
Behavioral biometrics
AI-based data security systems track behavioral biometrics, such as keystroke dynamics, mouse movements, and browsing patterns, to verify users’ identities or identify imposters. This form of continuous authentication ensures that access to sensitive data is granted only to legitimate users, enhancing security without compromising user experience.
Data loss prevention (DLP)
AI-powered DLP solutions monitor and control data transfers, preventing sensitive information from leaking outside an organization. By understanding the context and classification of data, AI in data security can enforce policies more accurately to minimize the risk of accidental mishandling of sensitive data or malicious data breaches.
Fraud detection
AI in data security used by the financial sector is broadly deployed to detect and prevent fraud. AI algorithms learn from historical transaction data to identify patterns and anomalies. These data security systems examine transaction patterns to identify unusual activities that could indicate fraudulent behavior (e.g., identity theft and unauthorized access to accounts).
Intrusion detection systems (IDS)
AI-powered IDS can learn and evolve to recognize new types of cyber threats by analyzing network traffic and system activities. Unlike traditional IDS, which rely on known signatures, AI-based systems can detect zero-day vulnerabilities and advanced persistent threats (APTs) with advanced behavioral analysis.
Phishing detection
AI enhances email security by identifying phishing attempts more effectively and accurately than conventional filters. By analyzing email content, metadata, sender information, and users’ behavioral patterns, AI in data security systems for email protection can flag suspicious emails that might otherwise bypass traditional security measures, thereby protecting sensitive information from being compromised.
Privacy protection
By automating data discovery and classification, AI supports effective data governance and privacy management. AI in data security helps identify and classify sensitive data within an organization’s systems to ensure that the proper protections and controls are in place and that they meet requirements for regulatory compliance.
Secure authentication
AI in data security tools enhances authentication methods by leveraging facial recognition, voice recognition, and other biometric authentication factors to provide secure and user-friendly access control. These AI-driven systems can adapt to changes in users’ biometric data over time to maintain a high degree of accuracy in user identification.
Security operations center (SOC) automation
With AI, many routine but important security tasks in security operations centers can be automated (e.g., log analysis and alert triaging by the SOC team). This improves the efficiency of SOC operations by leveraging powerful AI capabilities where they excel and freeing teams to focus on activities where human intervention is more valuable.
Vulnerability management
AI in data security tools can use specialized algorithms to scan networks and systems to identify vulnerabilities (e.g., outdated software or misconfigurations). By finding and prioritizing vulnerabilities based on threat levels, such as likelihood, scale, and potential impact, AI enables organizations to focus remediation efforts more effectively.
AI in Data Security Challenges and Limitations
For all of its benefits, AI in data security has challenges and limitations, including:
- Accuracy of AI in data security is contingent on the quality of the data ingested.
- AI-based security systems are targets for sophisticated cyber attacks aimed at exploiting system vulnerabilities or manipulating decision-making processes.
- Inaccurate threat assessments can result from biased or insufficient training data.
- Complexity and evolving nature of cyber threats require constant updating of AI models to maintain efficacy over time.
- Ethical and privacy concerns arise regarding the use of AI in monitoring and analyzing sensitive data.
- Expanded attack surface due to the vast amounts of data AI tools gather, store, and process.
- Human review and issue triage are often required when complex AI findings are broadly leveraged by security teams.
- Significant computational resources and expertise in cybersecurity and AI complicate its implementation.
- Skills gaps make it difficult for smaller organizations to reap the full benefits of AI.
Future Trends and Outlook on AI in Data Security
AI in data security is expected to continue to power more sophisticated, integrated, and proactive defense mechanisms against an evolving landscape of cyber threats. The following are five trends to look for:
- Advancements in machine learning algorithms will enable more precise predictive analytics to optimize early detection of threats and vulnerabilities before they are exploited.
- AI will become more autonomous and capable of making real-time decisions to counteract cyber threats preemptively.
- As IoT devices proliferate, AI will play a crucial role in securing IoT-based networks.
- Quantum computing is on track to enhance encryption methods significantly, making encrypted data virtually impregnable.
- The integration of AI with blockchain technology could revolutionize data integrity and authentication processes.
AI in Data Security a Must-Have
The integration of AI in data security brings enhanced intelligence and adaptive capabilities to cybersecurity preparedness. AI’s inherent strengths are dramatically improving the efficacy of threat and vulnerability detection, while enabling automation and precision actions that allow organizations to prevent, respond to, and mitigate potential cyber threats. As AI technologies continue to evolve, they promise to bring more advancements to fortify data security defenses even further. The solutions available today should be adopted and integrated into cybersecurity postures to meet data protection requirements.
Egnyte has experts ready to answer your questions. For more than a decade, Egnyte has helped more than 22,000+ customers with millions of users worldwide.
Last Updated: 18th April, 2024
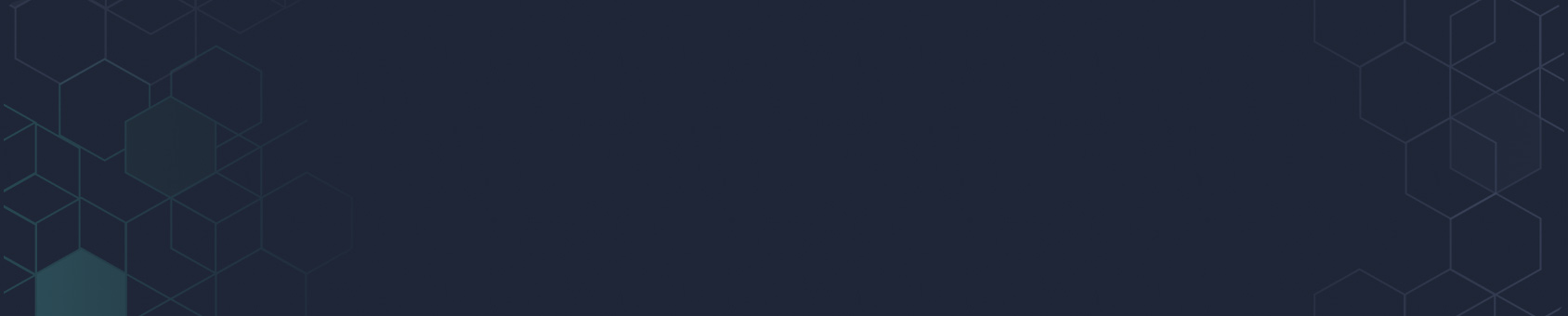