Data classification is a data management process used to sort and categorize information assets. Categorization protocols are applied to data types and data sets.

The primary driver for data classification is the protection of sensitive information, which is categorized according to confidentiality level. Confidentiality levels direct storage requirements and provide guardrails for usage and access. A close second reason for data classification is to make it faster and easier to store and retrieve information.
Data classification allows organizations to more safely and effectively manage information assets that are often spread across on-prem and cloud applications as well as different locations, such as network servers, folders, and hard drives. Sensitive information is tagged so it can be quickly be identified and organized appropriately to ensure its security.
Data classification benefits include:
- Providing a simple way to identify the level of security and privacy protection to be applied to a data type or data set to ensure that the right access controls are enforced
- Reducing the likelihood of a data breach or other type of cyberattack by improving the management of sensitive information
- Making data easy to find and retrieve so it can be used effectively and efficiently
- Optimizing adherence to risk management, compliance, and legal requirements
- Unlocking the full potential of its data to drive innovation and productivity
- Establishing what data there is, where it is stored, and how valuable it is
- Identifying what can be archived or deleted to reduce storage and retention costs
Let’s jump in and learn:
Why Data Classification is Necessary
Data classification is necessary for organizations to optimize data security, provide fast and easy access to data, and maintain data integrity. A well-planned system also supports risk management, legal discovery, and compliance. Data classification also plays a critical role in three key areas: security, compliance, and operations.
Improved Security and Confidentiality
- Safeguard sensitive corporate and customer information with access controls and encryption protocols
- Gain visibility into what sensitive information is in systems and where it is stored
- Know who has access to data, who can modify it, and who can delete it
- Support data retention programs to reduce the sensitive data footprint
- Understand the impact if the data is leaked, destroyed, or tampered with
- Eliminate data protection redundancies
- Streamline and strengthen data privacy and security programs
- Categorize unstructured data to lower the risk of unstructured sensitive information being compromised
Compliance
- Ensure that data is traceable and searchable
- Enforce rules for handling sensitive data according to compliance requirements
- Maintain day-to-day compliance as new data is created
- Retrieve data within timeframes set forth in regulations
- Be prepared for compliance-related audits
Enhanced Operation Efficiency and Reduced Risk
- Ensure that data is effectively protected, stored, and managed
- Improve data visibility, insight, and control
- Enable more efficient access to and use of sensitive information
- Support risk management efforts by making it easier to assess data’s value and the impact of improper access and use
- Simplify and expedite record retention and legal discovery
Data Classification Types
Data classification types fall into three approaches, each with its own techniques, benefits, and limitations. The three data classification types, with regard to approach, are content-based, context-based, and user-driven (sometimes called user-applied). Most organizations use a combination of these types.
- Content-Based
With content-based classification, files’ content is automatically inspected to assess sensitivity—eliminating end-user involvement. - Context-Based
Context-based data classification determines sensitivity based on indicators, such as application, users, location, and creator. - User-Driven (user-applied)
User-based data classification relies on users to manually specific sensitivity based on their discretion and subject matter expertise.
Data Sensitivity Levels
Standard data sensitivity levels differ slightly between business or organizations and government. These are associated with data types and data sets and are used to identify the various security and privacy standards that should be applied to data.
Whether in business or government, personal information must be protected according to protocols and rules for proper use. Laws protect much of the personal data held by a business or government entity.
Data Sensitivity Levels Used by Businesses
- Restricted: Highly sensitive data have restricted access and use, usually limited to “on a need-to-know basis.” Restricted data includes intellectual property, trade secrets, personally identifiable information (PII), cardholder data, and health information. Disclosure would trigger significant financial or legal impact on the business.
- Confidential: Data considered confidential is used across an organization, but it needs to be contained within the business. Confidential data may also have legal restrictions related to how it is handled. Examples of confidential data are pricing, marketing plans, or contracts. Disclosure of confidential data could negatively impact operations and brand.
- Internal: Information that is available company-wide is considered internal data that requires limited protection. Examples of internal data are company directories, employee handbooks, and company-wide memos. Disclosure of internal data will have a minimal impact.
- Public: A public data classification means that the information can be openly shared and does not require security controls when stored or used. This information can be freely shared with the public without any repercussions.
Data Sensitivity Levels Used in Government
- Top Secret: The highest levels of protection and access is used for top-secret data, which is restricted to persons with a “need to know.” If disclosed, top-secret data would be a grave threat to national security.
- Secret: This data is given a high level of protection. If disclosed, secret information would cause serious damage to national security.
- Confidential: The lowest level of government classified data, confidential data, requires far less protection than secret or top-secret data. If disclosed, confidential information would cause some harm to national security.
- Sensitive but Unclassified (SBU): Data that is not classified, but should be protected from release, is categorized as sensitive but unclassified. If disclosed, SBU data could violate the privacy rights of citizens.
- Unclassified: Unclassified is data that is not sensitive and requires no protection.
Data Classification Examples
To better understand how data classification instantiates itself in the “real world,” it helps to review an example. The following is from a medical institution’s data classification reference guide.
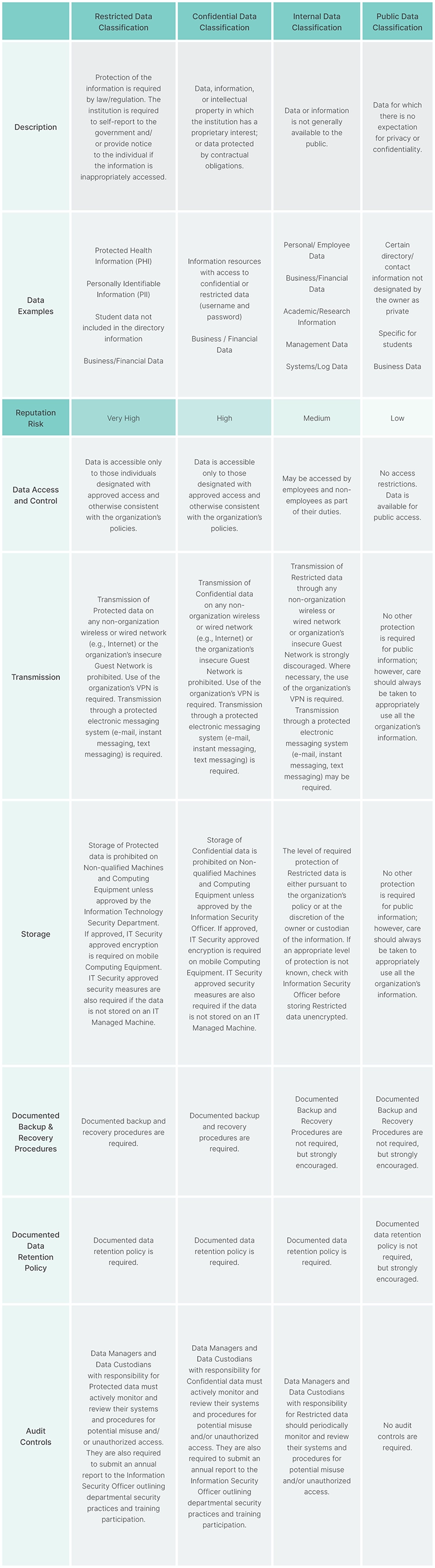
The Data Classification Process
When thinking about data classification, it is important to focus on why it is being put in place. Data classification is inherently complex, but order and process will help make this project go more smoothly and yield optimal results.
Critical steps in the data classification process should be considered in advance of starting a project. After securing buy-in from the organization’s leadership team and key constituents, these critical steps will guide the process for setting up and maintaining an effective program.
Getting Started with Data Classification
- Establish the criteria and categories for classifying data and develop a data classification framework and rules
- Find the data—all of it—then sort it according to the level of sensitivity and protection priority
- Understand what compliance requirements the organization must follow
- Put in place security protocols for each protection level
- Define employees’ responsibilities and roles in the initial process
- Implement various security-related measures based on the results of the classification process
- Employ best-of-breed solutions for automation, management, and monitoring
- Create an oversight team to ensure that proper protocols are followed
Data Classification Requirements
Five Success Criteria
- Provide clear, easily consumable procedures and guidelines.
- Set up guardrails to ensure that users follow data classification processes.
- Train everyone on how to use data classification protocols and follow rules.
- Specify employees’ roles and responsibilities as related to data stewardship.
- Define data lifecycle requirements as part of the security directives.
Data Classification Steps
Before starting with a data classification project, prioritize the data the needs to be classified. This is important, because not all data needs to be classified. More often not, data needs to be destroyed to reduce both clutter and risk. Also, take privacy requirements into consideration.
Three Steps to Prepare Data for Classification
- Scan
Take stock of all of the data to help direct the formation of a data classification plan that is in line with organizational goals and the data. - Identify
Find all data identifiers that can be used to organize data into searchable, sortable categories, such as file type, location, creator, character units, and size of data packets. - Separate
Organize the data according to category and identify sensitive data. Then sort it according to sensitivity, so the most sensitive information can be protected quickly.
Five Steps to Prepare Data for Classification
- Assess risks
Confidentiality and privacy requirements should be clearly understood from the start. - Develop classification policies
Create a data classification policy document that can be easily understood and followed by all employees. - Establish data categorization criteria
Understand what data is being organized, the importance of each bit, and the priority for categorization. - Find the data
Identify not just where the existing data resides, but where newly created data will come from to ensure that policies cover all data. - Set up and maintain security protocols
Ensure that data has the correct protection based on its category and that there are processes in place to update security protocols regularly.
Once structured data has been categorized, unstructured data should also be organized and categorized. This not only protects against the inherent risks associated with unstructured data, but also makes it more accessible, so the organization can extract value from it.
Data Classification Best Practices
Taking time to follow these data classification best practices will mitigate issues and make the process run more smoothly with better results.
- Identify where critical IP, sensitive information, or regulated data resides—check all possible locations, including hard drives, databases, network files, folders, cloud applications, and external storage
- Define core data categories—best to start with the basics, then add layers later
- Find the most important and valuable data—take advantage of technology to automate and manage data classification
- Train existing and new employees—remember the importance and efficacy of providing training and accessible resources
- Know the rules—understand the corporate, industry, and government requirements for data and use data classification to avoid non-compliance
- Engage end-users—create a system that users can understand and be comfortable using.
Data Classification Myths
Here are three truths that debunk the most common data classification myths.
- Data classification does not have to be highly complicated—start with a few categories and keep going.
- Data classification does not lead to unnecessary bureaucracy. Rather, it simplifies data protection by automating the process seamlessly for end-users.
- Data classification pays off from day one, with the value delivered growing over time, and the opportunity for even more benefits is substantial.
Widely held misconceptions about data classification should not get in the way of getting started on a program. It is well worth the investment at any level that it is implemented.
Egnyte has experts ready to answer your questions. For more than a decade, Egnyte has helped more than 16,000 customers with millions of customers worldwide.